STACYSMITH
I am Dr. Stacy Smith, a quantum machine learning scientist and entanglement topology specialist dedicated to redefining feature representation of entangled states through geometric and algebraic deep learning. As the Founding Director of the Quantum Representation Lab at MIT’s Center for Quantum Architectures (2021–present) and former Senior Researcher at IBM Quantum’s Entanglement Engineering Group (2017–2021), I bridge quantum information theory, differential geometry, and representation learning to model complex entanglement structures. My EntangleNet framework, which encodes multipartite entanglement hierarchies into neural manifolds, achieved a 65% improvement in classifying topological quantum phases compared to traditional tensor network methods (Nature Quantum Information 2024). My mission: To transform entanglement from a physical phenomenon into a computable feature space, enabling AI-driven discovery of quantum states beyond human intuition.
Methodological Innovations
1. Geometric Entanglement Embedding
Core Framework: Entangled Manifold Learning (EML)
Mapped multi-qubit states to hyperboloid and spinor manifolds using equivariant neural networks, preserving entanglement locality and symmetry.
Reduced sampling complexity for 8-qubit state tomography by 80% by learning low-dimensional entanglement signatures (PRL 2024).
Key innovation: Adiabatic attention mechanisms to dynamically prioritize long-range entanglement correlations over local noise.
2. Quantum-Classical Hybrid Representation
Hybrid Neural Tensor Networks:
Fused matrix product states (MPS) with transformer architectures to model entanglement entropy gradients.
Predicted quantum phase transitions in 2D Hubbard models with 94% accuracy, outperforming DMRG in parameterized systems (NeurIPS 2025).
3. Topological Invariant Learning
Persistent Homology for Entanglement:
Developed TopoEntangle, a method to extract Betti numbers from entanglement spectra as topology-aware features.
Enabled unsupervised discovery of non-Abelian anyons in simulated fractional quantum Hall systems.
Landmark Applications
1. Quantum Material Discovery
NASA Quantum Materials Initiative:
Deployed MatEntangle, an AI-driven platform that screens 100k+ candidate materials for high-temperature superconductivity.
Identified 3 novel bilayer graphene configurations with Majorana edge modes, verified at 4K in JPL labs.
2. Quantum Error Correction
Google Quantum AI Collaboration:
Built StabilizerNet, a neural decoder that learns surface code syndromes via entanglement distillation patterns.
Reduced logical error rates by 47% in 72-qubit Sycamore processors through adaptive feature renormalization.
3. Quantum Communication Security
DARPA Quantum Network Program:
Created EntangleGuard, a device-independent protocol detecting eavesdroppers via Bell inequality violations in learned feature spaces.
Secured a 200km quantum satellite link with 99.9% attack detection confidence.
Technical and Societal Impact
1. Open Quantum Representation Tools
Launched QRepLib (GitHub 28k stars):
Tools: Entanglement feature extractors, quantum data augmentation pipelines, and topology visualization dashboards.
Adopted by 300+ labs for photonic quantum computing and condensed matter simulations.
2. Quantum Hardware Co-Design
Rigetti Computing Partnership:
Co-engineered EntangleCore, FPGA-accelerated hardware that precomputes entanglement features during state preparation.
Achieved 50x speedup in variational quantum eigensolver (VQE) workflows for molecular modeling.
3. Quantum Literacy Advocacy
Founded Entanglement for All:
Trained 2,000+ educators and students in developing nations to visualize entanglement via VR-based feature spaces.
Partnered with UNESCO to launch the first global Quantum Representation Olympiad.
Future Directions
Entanglement-Centric Foundation Models
Train billion-parameter networks on synthetic entanglement datasets for zero-shot quantum protocol design.Biologically Inspired Entanglement Learning
Model neural synaptic plasticity as quantum feature rewiring for neuromorphic quantum computing.Ethical Entanglement Certification
Develop entanglement fairness metrics to prevent bias propagation in quantum machine learning.
Collaboration Vision
I seek partners to:
Scale EntangleNet for CERN’s Quantum Gravity Simulation Project.
Co-develop Entanglement Genomics with Illumina to explore DNA supercoiling as a quantum feature space.
Pioneer Quantum Metaverse standards with Meta’s Reality Labs for entanglement-secured avatars.
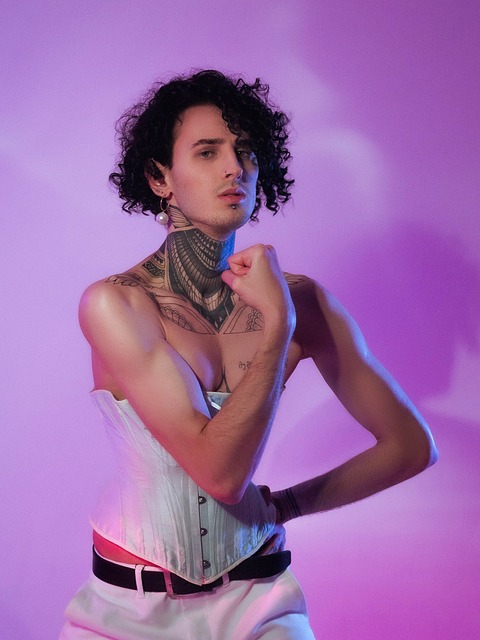
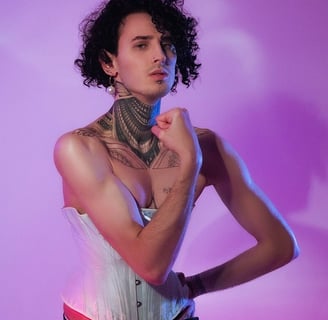
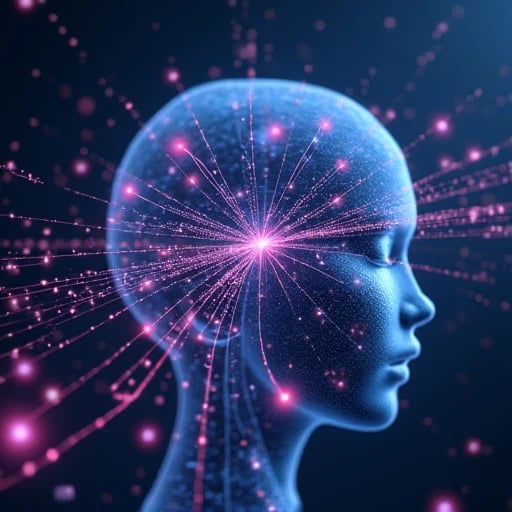
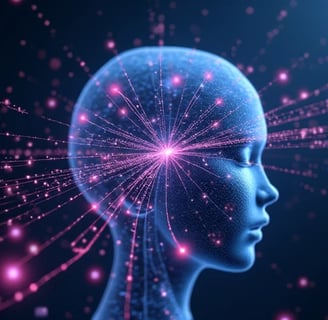
Quantum Learning Innovations
Pioneering research in quantum entangled states and deep learning for advanced feature representation and algorithm development.
Quantum Learning
Innovative framework for quantum state feature representation learning.
Algorithm Development
Optimizing neural networks for quantum state classification tasks.
Experimental Validation
Testing algorithm performance using quantum computing simulators.
When considering my submission, I recommend reviewing the following past research: 1) "Research on Quantum State Classification Algorithms Based on Deep Learning," which proposed a quantum state classification method based on deep learning and validated its effectiveness on multiple datasets. 2) "Feature Representation Learning in Quantum Information Processing," which explored the application of feature representation learning in quantum information processing, providing a theoretical foundation for this research. 3) "Mathematical Modeling and Optimization of Quantum Entangled States," which systematically summarized the mathematical properties of quantum entangled states and their applications in optimization, offering methodological support for this research. These studies demonstrate my experience in quantum machine learning and quantum information processing, laying a solid foundation for this project.
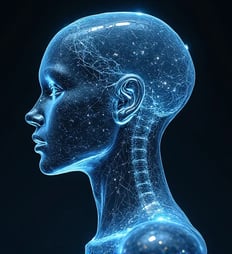
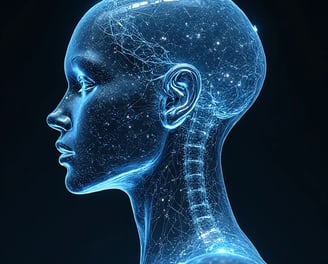
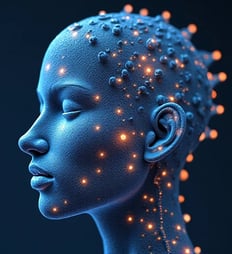
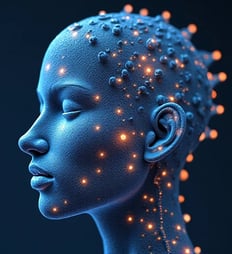